Instrumental Variables Explained: What are IVs and How are They Used in Econometrics?
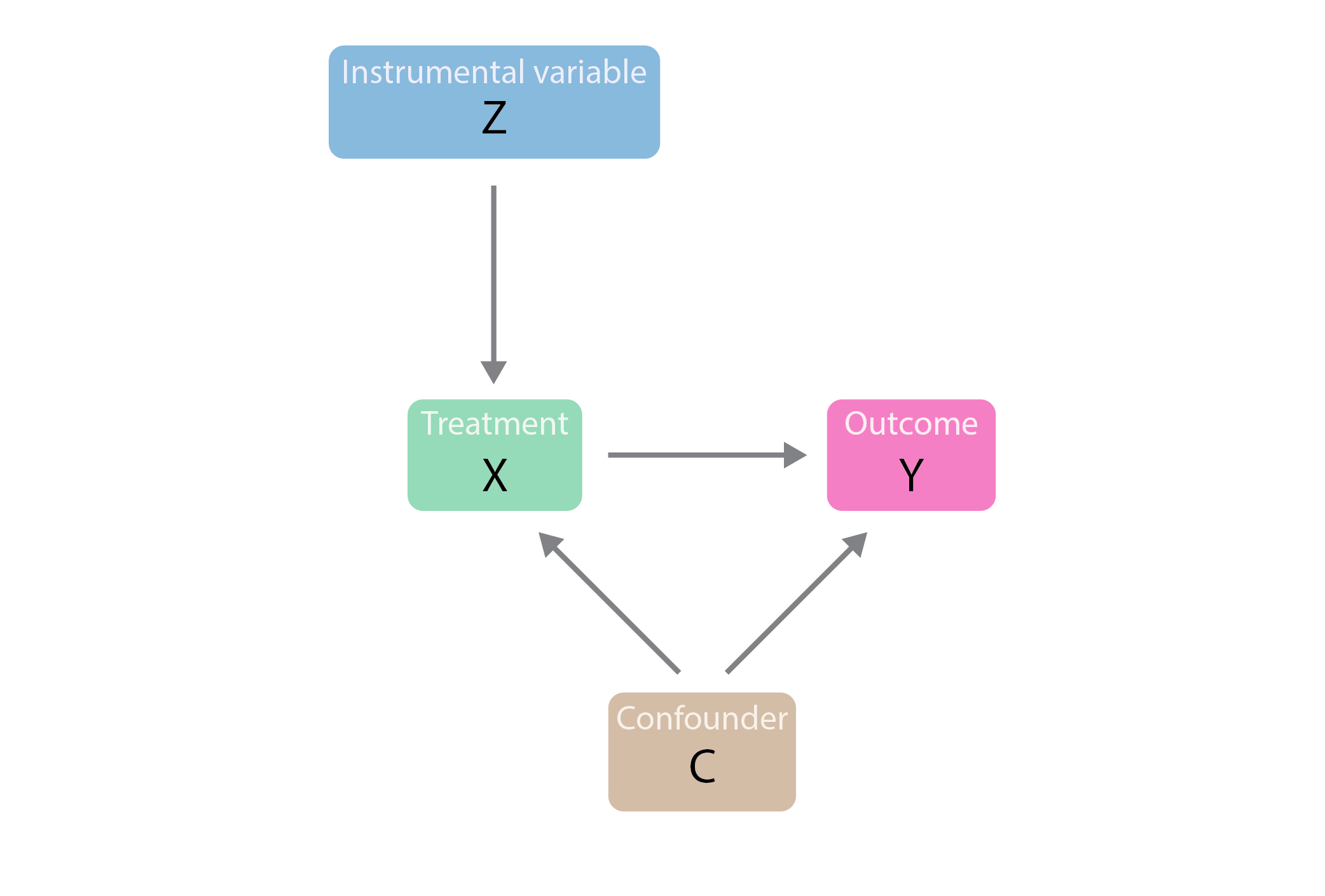
Overview
Intro:
- Definition of instrumental variables (IVs) - variables used to isolate exogenous variation in explanatory variables
- Brief background on why they are needed - endogeneity and inability to run controlled experiments
Section 1: Properties of Valid Instrumental Variables
- Relevance - correlated with explanatory variable
- Exogeneity - uncorrelated with error term
- Exclusion - does not belong directly in the explanatory equation
Section 2: Example of Using IVs
- A concrete example of a research question and endogenous explanatory variable (e.g. effect of education on earnings)
- Explain the proposed instrument (e.g. distance to college)
- Walk through the two-stage IV models, highlight the first stage and second stage
- Interpret the IV coefficient as a causal estimate of the effect of explanatory variable on the outcome
Section 3: Why Use IVs?
- Allow consistent estimation of causal effects in the presence of endogeneity
- Use exogenous variation from the instrument
- IV as a solution to omitted variable bias
Section 4: Challenges with IVs
- Finding valid instruments
- Weak instrument issues
- Interpreting coefficients
- Generalizability of estimates
Conclusion:
- Recap key points about what IVs are and why they are used
- Discussion of IVs' importance and limitations in econometrics
- Concluding thoughts on applying IVs appropriately
Introduction
Endogeneity is the bane of many an econometric analysis. Without properly accounting for it, researchers risk making erroneous inferences about causal relationships from observational data. However, instrumental variables (IVs) offer a sophisticated method to isolate exogenous variation in explanatory variables. Economists can circumvent endogeneity bias by leveraging instruments that provide quasi-experimental changes in predictors. This allows for consistent estimation of causal effects even in the absence of randomized controlled trials.
Instrumental variables(IVs) can be used to estimate causal relationships in cases where controlled experiments are not possible. IVs allow us to isolate exogenous variation in explanatory variables--allowing economists to estimate causal relationships. IVs are required because of a concept named endogeneity, where explanatory variables are influenced by other factors in the system. The existence of endogeneity in a model leads to biased and inconsistent estimates. Since controlled experiments are often unachievable in economics, IVs have become a crucial crutch for identifying causal effects.
Section 1: Properties of Valid Instrumental Variables
For an instrumental variable to be valid, it must satisfy three core properties:
Relevance
Relevance is the first core principle. The instrument variable must be correlated with the endogenous explanatory variable in the model. Intuitively speaking, the IV needs to be able to predict changes in the explanatory variable - as it cannot be weak or insignificant in the first stage regression. A strong relationship ensures that when the instrument varies, it creates ample exogenous variation in the explanatory variable.
Exogeneity
The instrument cannot be correlated with the error term in the main regression equation. A zero direct relationship is required between the IV and the outcome variable after controlling for the explanatory variable. This ensures changes in the instrument only affect the outcome through the explanatory variable channel.
Exclusion
The instrument must be excluded from the main regression equation. It cannot have a direct causal effect on the outcome variable - its role is limited to the first stage. The IV creates exogenous variation in the explanatory variable, which in turn affects the outcome.
Finding variables that satisfy relevance, exogeneity, and exclusion restriction is difficult in practice, but when valid IVS are identified, they allow an unbiased causal estimation of the explanatory variable's effect on the outcome.
Section 2: Examples of Using IVs
Let's walk through a detailed example to illustrate how IVs can estimate causal effects.
Research Question: What is the causal effect of years of education on earnings for men in their late 20s?
Endogenous Explanatory Variable: Years of education
This is endogenous because innate ability, family background, and motivation influence both education and earnings.
Proposed Instrument: Distance to nearest 4-year college when growing up
This satisfies relevance because living closer to college increases years of education. It satisfies exogeneity because after controlling for education, distance does not directly affect earnings. It satisfies exclusion because distance does not belong in the earnings equation.
Two Stage Model:
First Stage:
Ei = β0 + β1Di + β2Ai + β3Fi + ui
Second Stage:
Yi = γ0 + γ1Êi + γ2Ai + γ3Fi + vi
Where:
Ei = Years of education for person i
Yi = Earnings for the person i
Di = Distance to college for person i
Ai = Ability of person i
Fi = Family background of person i
Êi = Predicted education from the first stage
The coefficients γ1 show the causal effect of each additional year of education on earnings. The IV approach removes omitted variable bias and allows us to consistently estimate the true causal impact.
Section 3: Why IVs?
Instrumental variables are widely used simply because they allow consistent estimation of causal relationships, even when explanatory variables suffer from endogeneity. There are still other several key reasons why IVs are preferred in the field:
- IVs mitigate omitted variable bias and endogeneity bias. By leveraging exogenous variation from the instrument, the effect of confounding factors gets blocked and we can isolate the causal impact of the explanatory variable.
- IVs allow us to estimate causal effects, rather than just correlations. Most economic models estimate association but fail to claim true causality due to endogeneity. Properly applied IV methods let economists make causal inferences.
- IVs enable estimating the local average treatment effect(LATE). The IV estimate shows the causal effect specifically for the subpopulation whose treatment status is influenced by the instrument. This is informative for policy targeting certain groups.
- In the absence of controlled experiments, in real-world applications, IVs provide a feasible solution. Field/lab experiments are often costly or infeasible, but IV methods allow economists to simulate experimental conditions using observational data.
- IVs help evaluate policy interventions when randomized trials are unethical. For example, we cannot randomize education policies, but we can use the distance to school as an instrument instead.
In summary, IVs are a powerful econometric tool for determining causality rather than just correlations. By leveraging instruments that induce exogenous variation in the explanatory variables, economists can obtain consistent estimations of causal effects. This makes IVs invaluable in many empirical research settings.
Section 4: Challenges with IVs
While IVs can estimate consistent causal effects, there are many limitations and challenges involved with implementing IV methods:
- Finding valid instruments: The first and foremost problem is identifying instruments that satisfy the three properties: relevance, exogeneity, and exclusion restrictions. Invalid instruments will lead to biased estimates. Domain expertise is needed to justify instruments
- Weak instruments: Even if the instrument satisfies the properties, it may only weakly correlate with the explanatory variable. This again, will lead to imprecise estimates and biases towards OLS.
- Interpreting coefficients: IV estimates a local average treatment effect that only applies to the subpopulation affected by the instrument. The estimate may differ from the overall average effect. Clarifying what the LATE is is crucial.
- Generalizability: The local estimate from an IV analysis may not generalize well to the overall population. Out-of-sample predictions and external validity need to be addressed.
- Violations of assumptions: Like any method, IVs rely on certain assumptions being met. Violations such as direct effects or imperfect exclusion bias the estimates. Sensitivity analysis helps assess this.
Although IV methods sound like a golden key, they often require careful design, rigorous statistical testing, and transparent discussion of limitations.
Conclusion
In this overview, we have covered the theoretical underpinnings and practical applications of instrumental variables, a vital technique for causal inference in econometrics. When explanatory variables are endogenous, IVs allow researchers to isolate exogenous variation and obtain unbiased and consistent estimates of causal effects. Instruments must satisfy relevance, exogeneity, and exclusion restrictions to be valid.
However, finding appropriate instruments is challenging in practice. Even valid IVs estimate local treatment effects that may not generalize broadly. Violations of assumptions can undermine results. Thus, IV methods require rigorous statistical testing and transparent discussion of limitations.
Still, when properly implemented, IVs provide invaluable causal inferences that are simply not possible using conventional regression techniques on observational data. As such, instrumental variables should belong in the toolkit of any serious applied economist. By combining domain expertise for instrument selection with econometric rigor, researchers can employ IVs to disentangle complex causal mechanisms.
This capacity is crucial for empirically validating economic theories and guiding policy decisions. From labor markets to health economics, impact evaluations using IV stand at the frontier of establishing causality in economics. Though not without limitations, IVs represent a major methodological advance that empowers economists to tackle endogeneity bias and advance scientific understanding.